Context-Aware Implicit Feedbackbased Hotel Recommender System for Anonymous Business Travellers
- Molood Arman
- Nov 24, 2023
- 5 min read
Updated: Mar 23
In real world, mostly the ratings from users are not provided. Implicit feedback from historical booking data can be the only available data. This data is often very sparse, causing collaborative filtering techniques to decrease significantly in their recommendation performance. To solve the sparsity problem, side information (e.g. item or user profiles) can be utilized. Also, Matrix Factorization (MF) is an appealing recent method which can handle the sparsity problem, the latent user or item representations learned by gradient descent methods in MF can improve the recommendation performance. Sometimes, having access to the side information cannot be provided. One idea can be creating these profiles by extracting information from implicit data. In this master thesis, we built a hotel recommendation system for corporate travellers based on historical booking data. It is shown that we can improve the performance by using this kind of profiles as the content information and join them with collaborative filtering for the feedback information. Furthermore, post-filtering on aggregation of different contexts for having more accurate result can be applied to the model. Considering the OLAP architecture integrated by our final result from our model, we can contextualize them on different aggregation on contexts.
Problem Statement
For Business travelers, there is a travel policy among companies, travel agencies and Hotels. These travel policies are unknown to flight provider companies and they did not consider it in Hotel Optimizer. There are not any standards for these travel policies because each Travel Management Companies (TMC) defines their own policies to work with different companies and with different hotel chains in different cities. The main step for providing good recommendations for business travelers should be understanding these unknown business policies.
Traditional recommender systems operates in the two-dimensional User × Item space; because of that, they make their recommendations based only on the
user and item information and do not take into the consideration additional contextual information that may be crucial in some applications. However, in many situations the utility of a certain product/service to a user may depend significantly on other parameters like time (e.g., the time of the year, such as season or month, or the day of the week). It may also depend on the person with whom the product will be consumed or shared and under which circumstances. In such situations it may not be sufficient to simply recommend items to users; the recommender system must take additional contextual information, such as time, place into consideration when recommending a product [Adomavicius 2005b]. For example, when recommending hotels to a traveler, the system should also consider the place of those hotels to be in the same city as destination city of the traveler, budget and time can be other contexts which can be considered as traveling conditions and restrictions and many other contextual information. Here, for each traveler, the relation of the company, travel agency and the hotels which has the contract with that travel agency should be taken into account. It is important to extend traditional two dimensional User × Item recommendation methods to multidimensional settings. In addition, in several researches, it was shown that considering knowledge about users into the recommendation algorithm in certain applications can lead to better recommendations [Herlocker 2000]. To that end, this thesis focuses to design a hotel recommendation system for the anonymous business travelers when they reserve a flight. They are anonymous because even in the booking data, the company which booked these hotels are not mentioned. Also, gathering information about companies without their permission is not allowed. Then, the first step should be applying a method to find the related company to each transaction. Since our target group is business travelers, we should consider a group of customers from the same company as one user. Then actually for finding a user, we have to cluster transactions from the same company in one group.
Also, the only feedback which we have about interest of companies to book hotels are derived from historical data of booking and we do not have access to the direct ratings of users about hotels. Then we should use the methods which have a better performance for implicit feedback data.
Objectives
The final approach which we implement to reach these goals for this project guides us to these deliverables:
Developing a model-based recommendation system which can work just based on implicit data;
Creating the profiles per each user and item by just using the implicit data and the interaction of the users with items based on the transactions data;
Having a better performance by embedding these created profiles in the learning process of model-based recommender system;
Considering context to give more accurate recommendations to each customer.
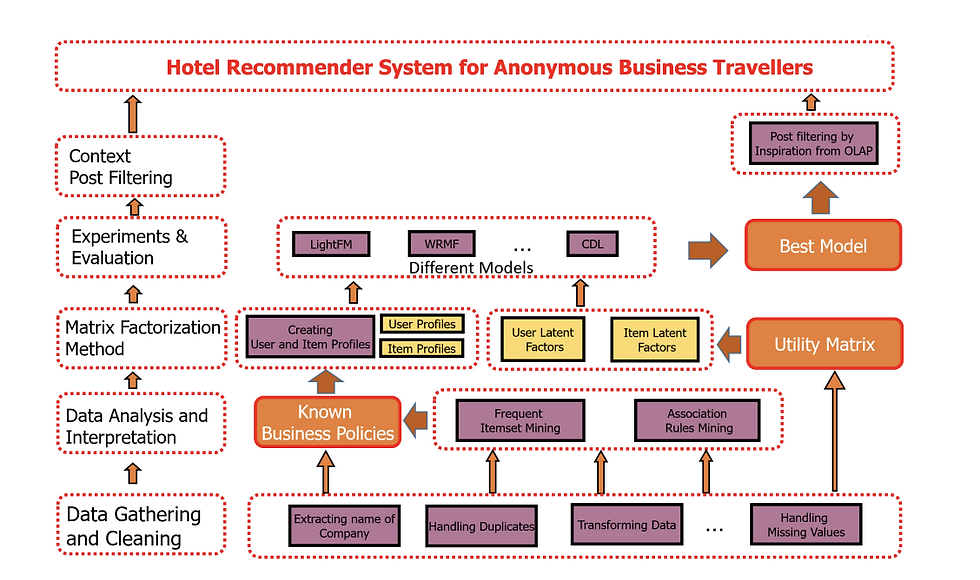
Methodology to build a hotel recommender system
The Original Dataset :
The data consists of:
Implicit feedback from music listening behavior, spanning several thousand users (companies) and several thousand Items (hotels) ( ~38,308 users, ~900,812 interactions, ~94,074 hotels). Each observation consists of a single interaction between a user and a hotel. Gathering Implicit feedback from users to create the utility matrix can be done in different way. The binary preferences of booking or not booking hotels by the user in the past can be considered as the implicit feedback, or the total number of the bookings of each hotel by each user in the past and normalize or scale the values. we chose the latter one. If we consider the whole number of users and Items, we have the density of 0.025% which is too low (0.025% of utility matrix space is filled). In this case, even thinking about applying collaborative filtering techniques which are based on finding similarity between items or users cannot have a good result. For decreasing the sparsity, I decided to consider the users which made the booking more than 5 times and the hotels which booked more than 5 times to have a warm start to build a recommendation system. In this case, we have the density of 0.178% which is not that much better but is less sparse. Collaborative filtering still cannot have a good result in this case, then we should think about other methods.
Implementation:
LightFM was introduced for the first time by Maciej Kula (who was working for Lyst - a fashion shopping website) in [Kula 2015]. LightFM is written in Cython and is paralellized via HOGWILD SGD (an update scheme to Parallelization Stochastic Gradient Descent). He released a Python implementation of LightFM and made the source code for his paper and all the experiments available on Github . For embedding the features and contextualizing them, I used LightFM method as the core of my algorithm and I added some extra functionality to the main method.
The code for this project is available publically here.
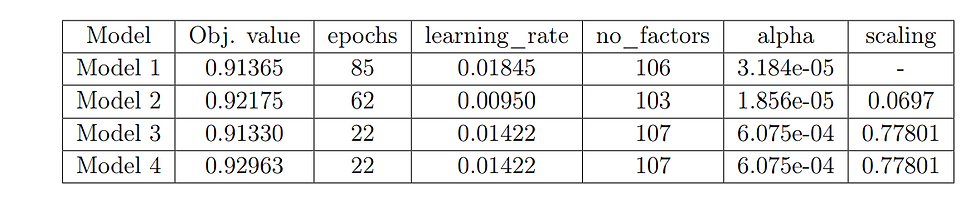
Hayperparameters for different LightFM models for WARP algorithm for evaluation dataset
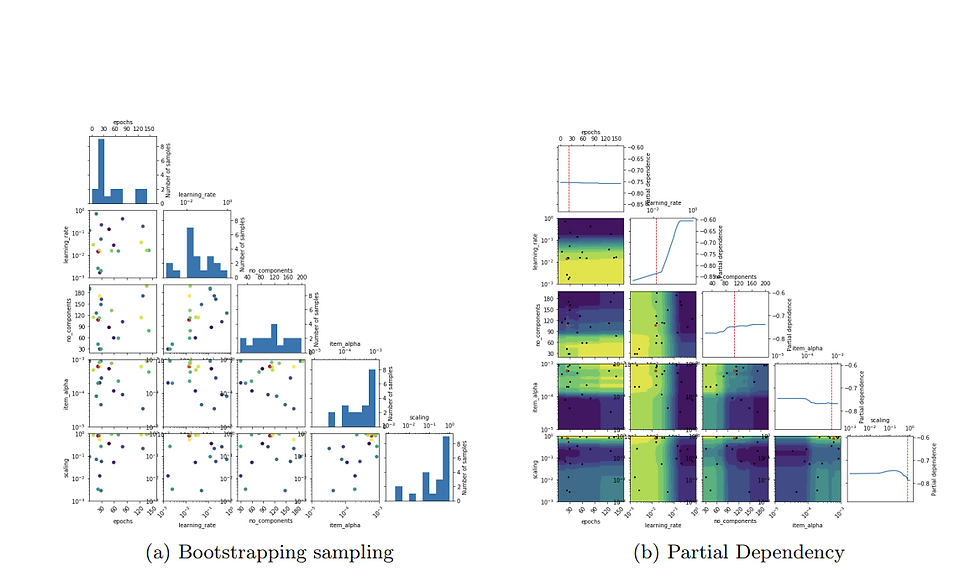
Hyperparameters selection by bootstrapping sampling for WARP algorithm for Model 4 (final model)_considering AUC as the objective function
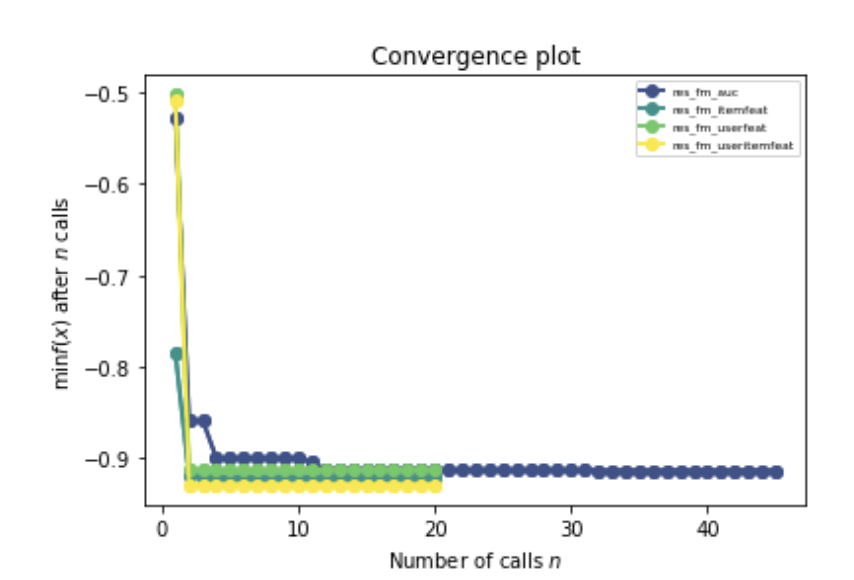
Comparison of AUC based on forest minimizing method for the 4 different model_WARP algorithm

Hayperparameters for different LightFM models for BPR algorithm for evaluation dataset
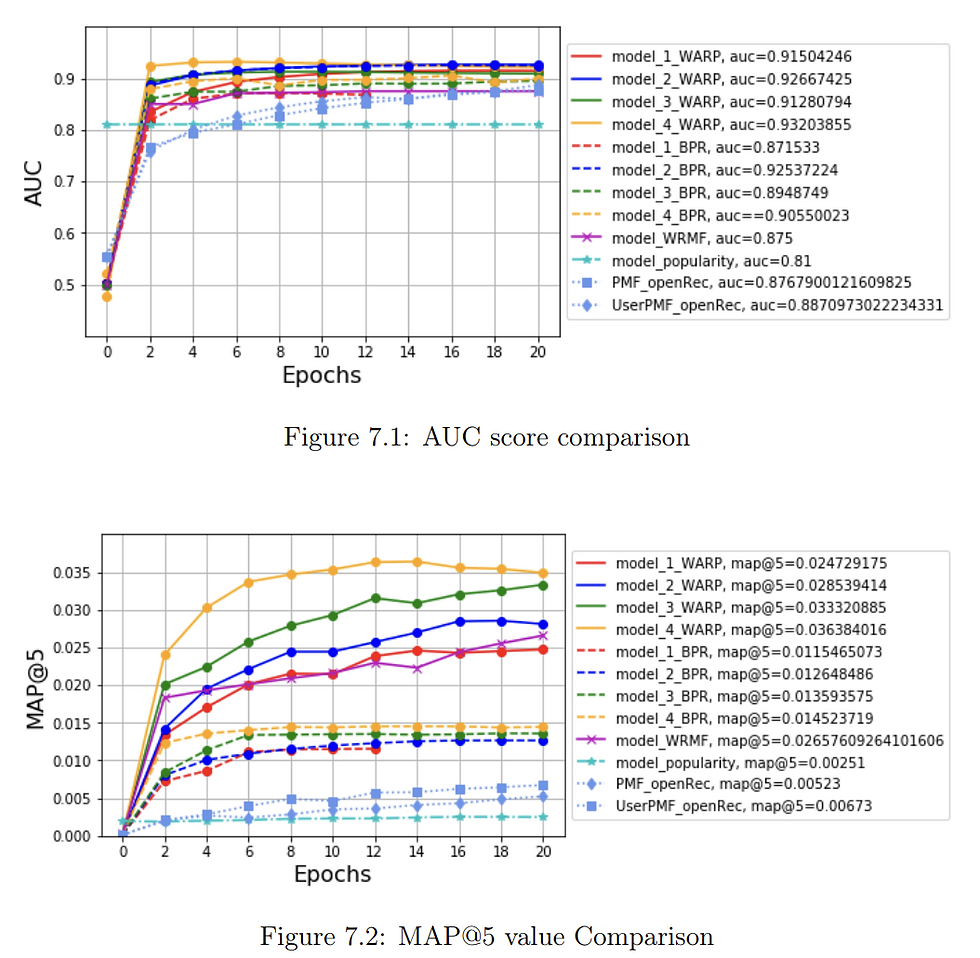
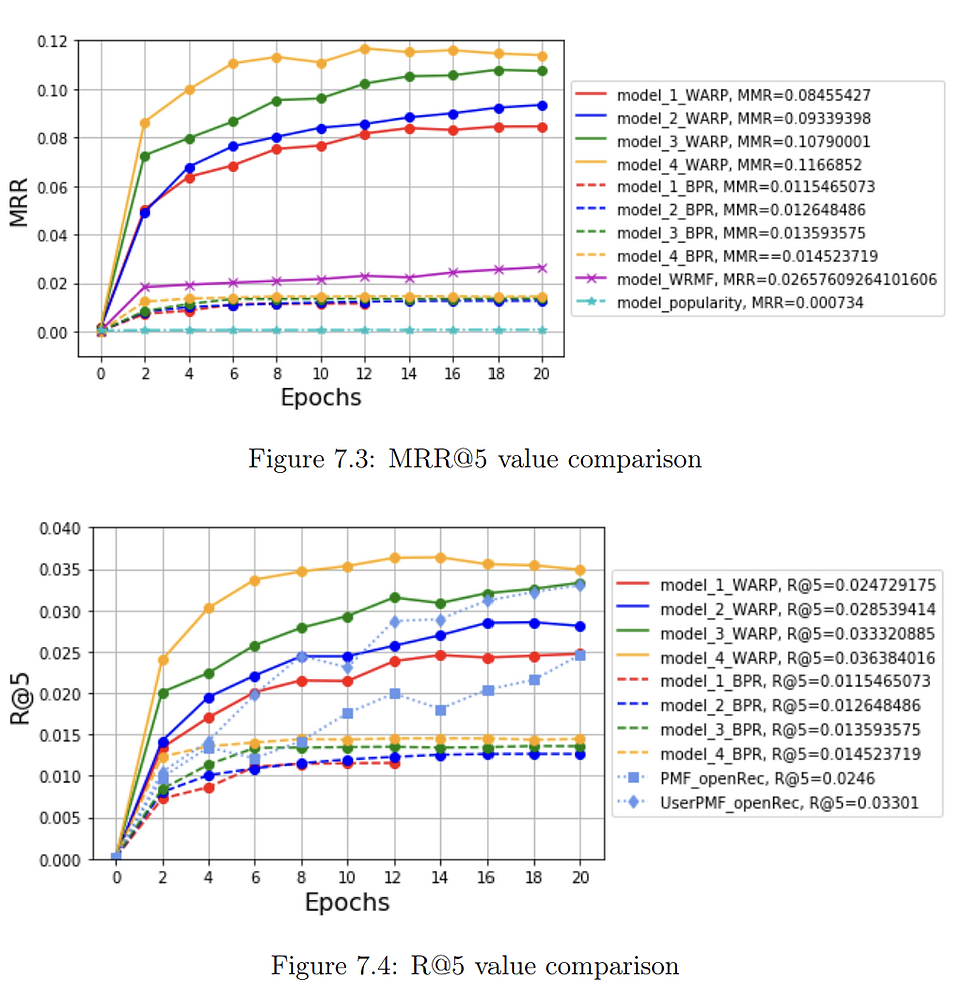
Comments